Here’s how to pick the right speech-to-text provider for your Speech AI journey
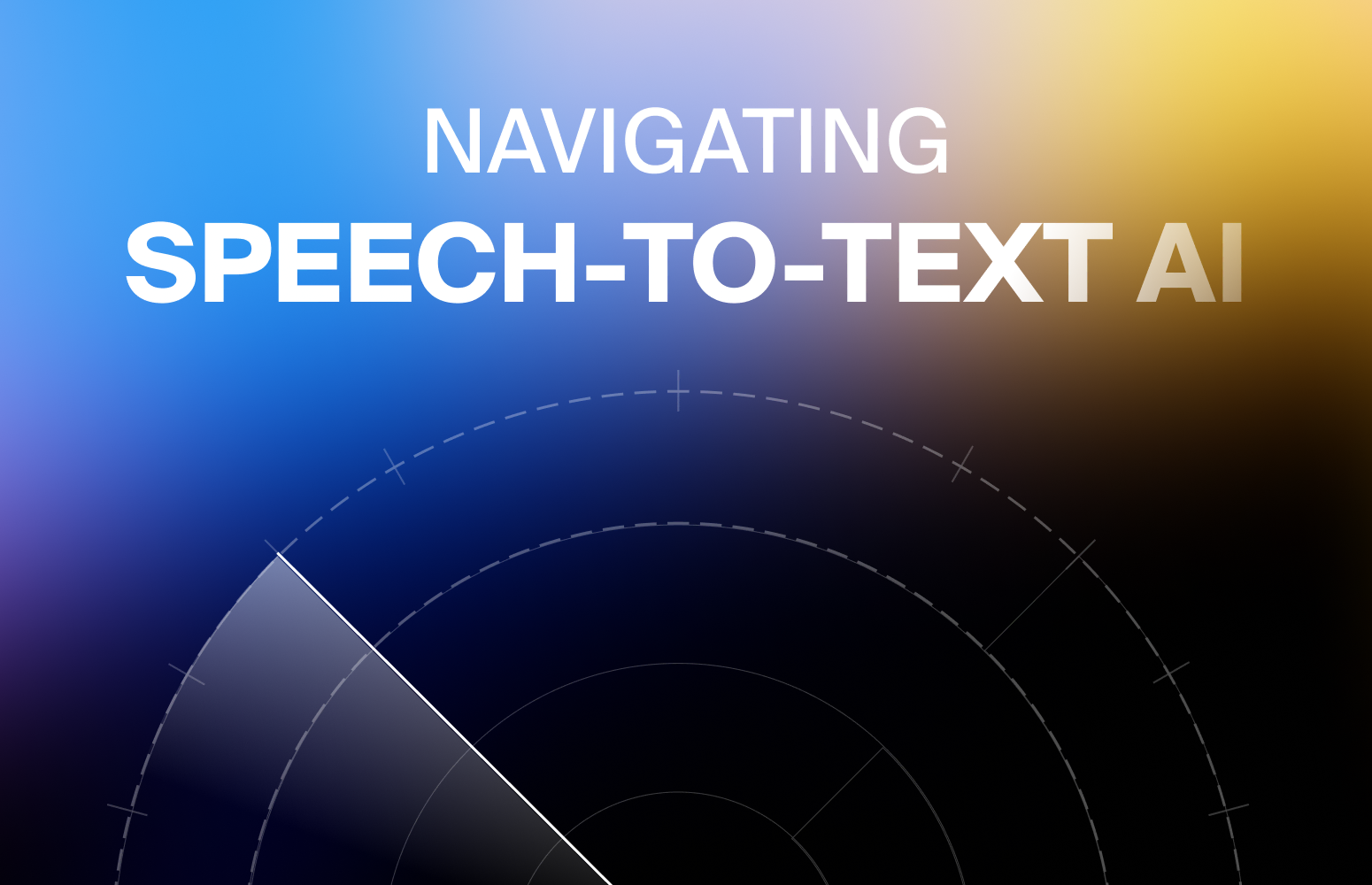
Until recently, AI speech-to-text has been reserved for the happy few. But commodification is on its way. As prices dropped while the accuracy and speed of transcription increased, there has been an explosion of speech-to-text providers catering to a broader range of companies and use cases. In this article, we give you a bird's-eye view of the market and introduce you to the speed-accuracy-cost tradeoff in audio transcription to help you pick the best Automatic Speech Recognition (ASR) provider for your use case and budget.
From niche to mainstream: the speech-to-text technology adoption curve
Did you know that airline fares have fallen by 50% since 1978?
If you’re wondering why we’re starting an article about the commodification of ASR technology by talking about air travel, just bear with us. It’ll all make sense in a bit.
Plane travel used to be reserved to the privileged few. There were a handful of major airlines (e.g. PanAm, Air France, Lufthansa, Alitalia, Iberia) who held quasi-monopolies on air travel and were thus able to provide pretty much the same (one-size-fits-all) service.
Yet faced with growing customer demand, custom options evolved. Suddenly, you could purchase a first class ticket, enjoy a custom menu while in the air, or upgrade to fast-track boarding.
Not only did the amount of options available to air travelers increase — airlines also began outcompeting each other to offer lower prices. As a consequence, since 1978, airline fares have declined drastically.

The airline industry offers an apt analogy for the state of the current ASR market.
Before the emergence of deep learning and neural networks in the 2010s, a handful of dominant players held a quasi monopoly on speech-to-text technology.
In the early 2000s, companies like Nuance Communications (now Nuance AI) owned expensive software programs like Dragon NaturallySpeaking, which was considered to be the best the market had to offer. Next, Big Tech providers like Google, Amazon, and Microsoft joined the market with their cloud-based APIs.
Yet, in the 2010s, the availability of open-source frameworks and increased research activity, led to a more accessible and competitive landscape for speech-to-text technology. Suddenly, the performance of ASR technologies skyrocketed due to more processing power and advances in Large Language Models (LLMs). At the same time, hardware costs are coming down — creating the perfect storm for widespread adoption and mass commodification.
Big Tech’s transcription services can be compared to the first airlines of the air travel industry. They were among the first to capture market share, and while they currently struggle to compete with new entrants who have disrupted the market with better, faster transcription and/or low-cost packages, they are able to stay afloat because of their first mover advantage and, crucially, because transcription services are just one part of a bigger bundle of services that they monetize.
The next wave of commodification
Today, in 2023, we’re at the cusp of the next wave of democratization: smaller, specialized providers who have made ASR their core business and are offering faster and cheaper solutions have entered the market — and they’re here to stay.
Just as Ryanair and EasyJet have made flying easily accessible to billions of people today, anyone with a wifi connection and a credit card can access transcription services for personal and business purposes.
And just like there are many types of airlines today, each catering to a specific segment of the air travel market, there are several STT providers to pick from — from the low-cost disrupters, to the middle-class favorites, and all the way to the “private-jet-like” hyper-specialized players.
This means companies looking to implement ASR solutions today have more choice than ever. Yet navigating the yet-to-mature market is far from easy. Amid all these options, how to choose the right one for your team and use case?
The speed-accuracy-cost tradeoff
Before diving into picking the right provider, we need to understand why the STT market has been traditionally underserved, and is currently so variable in terms of price and quality. After all, when we talk about engineering mastery in STT, we’re almost always referring to the speed-accuracy-cost tradeoff.
There’s an old saying: “you can have it fast and cheap, but it won’t be good; you can have it good and cheap, but it won’t be fast; or you can have it good and fast, but it won’t be cheap.”
This is true for many things, whether it’s buying your first home or learning a new language. It’s also true for ASR software.
In ASR, the speed-accuracy-cost tradeoff arises because optimizing one aspect often comes at the expense of another.
- Speed refers to how fast the algorithm can process and produce the speech recognition output. Using simpler models or reducing the computational complexity of the algorithms will speed up your transcription. However, this may result in lower accuracy as the system might struggle with handling complex or ambiguous speech inputs.
- Accuracy refers to how accurate the output is and the system’s ability to handle different scenarios, such as background noise, accents, and jargon. It’s key evaluation metric in ASR is word error rate (WER). Improving the accuracy of speech recognition typically requires more advanced models, larger datasets, and longer training times.
- Cost refers to how many resources (e.g. financial, HR, hardware) are required to develop, train, maintain, and run the system. Speed can be accomplished by investing in computational resources, such as faster processors or specialized hardware — with a significant price tag to follow.
Speed and quality are conflicting factors, meaning that improving one will compromise the other. The cost factor is a result of the choice made by the provider between speed and quality.
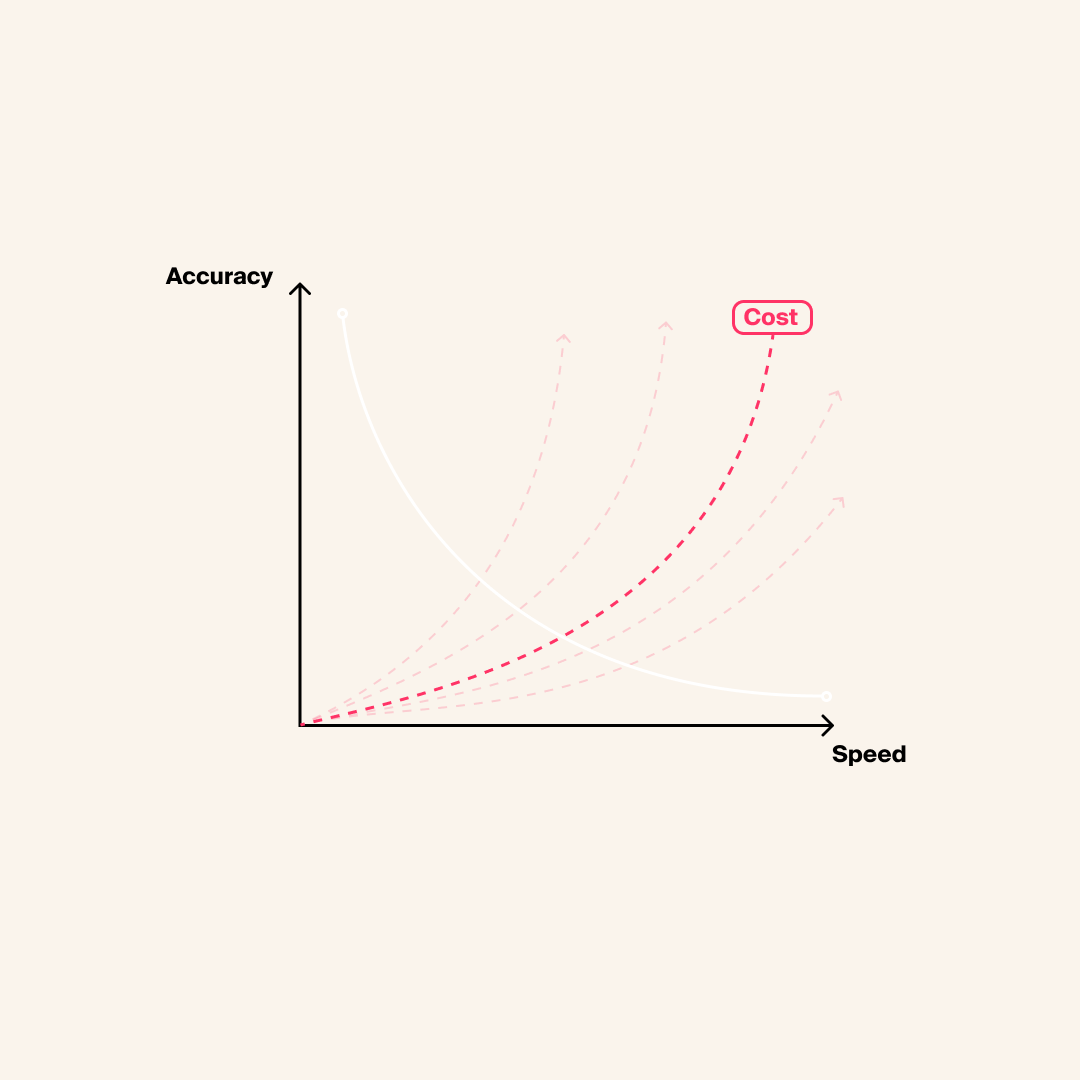
Let your use case determine your ASR provider
Whether or not you need hyper accurate speech-to-text transcription will depend on your use case.
For instance, media companies that rely on speech-to-text technology for video or podcast editing have little room for error. The same goes for subtitling or translation. Companies in these industries are often willing to sacrifice some speed for greater accuracy.
On the other hand, if you’re a call center, emergency service or financial trading firm, speed is the holy grail that enables you to serve your customers and stand out from your competitors.
Similarly, if you’re mainly looking to extract insights from recorded meetings, you’ll likely tolerate some errors in the summarization transcription as long as the main message still comes across. And if your main priority is ‘chapterization’ — breaking up lengthy videos into sections that are easily navigable — you’re more likely to tolerate a few errors in the transcription, too.
Wondering how your use case affects the price? Imagine your use case goes beyond basic transcription, requiring audio intelligence features, or specific jargon/ dialect to fine-tune models on. Then chances are that a one-size-fits-all service won't cover 100% of your needs.
Keep in mind that just because something is cheap (e.g. Ryanair), the extra costs and hidden fees (e.g. luggage add-ons, bus tickets to distant airports) could mean you might be better off paying for a more premium service – like Transavia or Air France, that give you a significantly better experience for a still reasonable cost.
When it comes to quality, with a basic transcription service (i.e. a low-cost “carrier” with no add-ons) you’re not always guaranteed to land as predicted. You might be rerouted to a random airport (i.e. due to technical errors) to load up on fuel, or experience lots of turbulence (e.g. due to hallucinations in the transcription) along your journey.
At the same time, choosing an expensive Big Tech provider isn’t a guarantee of success. If you require greater customizability or have a niche use case, these APIs are unlikely to match your needs. Furthermore, they tend to be far slower in terms of processing power, and produce less accurate transcriptions for a higher price.
Overall, the current ASR market can be expensive, non-transparent, and confusing. For startups and SMEs with tight budgets and ambitious use cases, picking the right ASR provider can feel like throwing darts while blindfolded — you might hit the bull’s eye, but you may very well miss the mark altogether.
The good news is, several providers today provide multi-destination, long-distance flights, covering the majority of needs and catering to a wide demographic. What changes is the price that’s being charged for a bundle of extra services; i.e. the number of destinations served.
While the basic transcription ‘engine’ is the same for everyone, ultimately, it is the engineering mastery of a specific provider that will determine which API will manage to meet the largest number of needs at the most affordable cost.
The sweet spot between speed, accuracy and cost: Gladia's approach
Since speed and quality in ASR are inversely proportional, a large neural network may be extremely accurate but take longer to compute, since the more computations and the more processing time and GPU power is needed. Conversely, a simple algorithm may bring quick results, but suffer from low accuracy.
To reconcile speed and quality, you can opt for a hybrid model that combines different architectures. This is also where the power of API providers like Gladia comes into play, since they can adopt various strategies to resolve the speed-quality dilemma. By striking a balance between speed and quality while offering customizable options, API providers can offer cost-effective solutions that cater to a wide range of user requirements.
At Gladia, we believe a good goal to strive for is to have an accurate transcription of one hour in under a minute. Using something that delivers results in 10 seconds but sacrificing quality is not desirable — but neither is waiting 20 minutes for a transcription of 1 hour.
The alpha version of Gladia’s API was extremely fast, with random errors (due to memory leaks) and an “ok” speaker separation (diarization), enabling a transcription of 1 hour in under 10 seconds, which affected the quality of the transcription.
While testing our product with early users and design partners such as call-centers, video and podcast platforms, we learned they expect to get results in under a minute, so we gradually improved the quality of the transcription by choosing a more precise algorithm with diarization and extra features to ensure utmost accuracy.
This brought down the speed, albeit slightly, not to mention requiring us to double down on hardware usage and GPU processing capacity, which brought up the cost — although not to a level that was unacceptable for our the many use cases we currently serve. Our pricing currently starts at $0.00017/sec, and is among the most affordable on the market given our multi-tier package. You can try it for free here.
Our metrics
Similar to airplanes, continuous improvement is deeply ingrained in our scientific DNA. When we encounter setbacks or failures, we embrace the opportunity to learn and implement new procedures.
In a recent study, it has become evident that relying solely on unit testing is insufficient. Instead, we have recognized the importance of incorporating additional metrics into our blocking unit tests, Word Error Rate (for accuracy), Diarization Error Rate (for speaker separation), Accumulated Average Shift or Mean Absolute Word Alignment Error (for word-level timestamp). By integrating these parameters, we aim to enhance the reliability and performance of our scientific processes.
Ensuring outmost quality
Currently, we have successfully addressed the quality aspect of our system, which has inadvertently led to a decrease in computational speed from 10sec (RTF 0.28%) originally to 50sec (RTF 1.39%). However, we are actively engaged in resolving this issue by focusing on improving the system's efficiency while maintaining the fixed quality standards. Our immediate goal is to achieve a 2-fold reduction in computation time (22sec/h, RTF 0.69%) within the next few weeks.
Additionally, we are exploring the potential for further optimization, particularly through key-value attention mechanism memory look-ups, which holds the promise of an additional 2-fold improvement in computational speed within a month (10-15sec/h, RTF 0.28%-0.42%). These scientific endeavors are aimed at striking a balance between computational efficiency and maintaining the desired level of quality in our system.
Final destination: speech-to-text for all
Just as airline carriers figured out how to distinguish their services from one another and cater to a specific subset of air travelers, the STT industry is on the cusp of a new wave of commodification that will lead to an explosion of customizable options and more affordable prices.
But our journey is not yet complete, and there will likely be turbulence on the way. You may experience hidden fees in your ticket, or unscheduled layovers at random airports. Or you might simply overpay for your ticket because you weren’t aware that cheaper alternatives existed.
Still, ten years from now, we’re betting STT technology will be as widespread and easy to access as commercial air travel. You’ll have the Ryanairs and Easyjets, the Lufthansas and the KLMs, and the Qatars of the audio intelligence industry — all serving their own unique customer segment.
Until then, we wish you a safe and comfortable journey.
About Gladia
At Gladia, we built an optimized version of Whisper in the form of an API, adapted to real-life use cases and distinguished by exceptional accuracy, speed, extended multilingual capabilities and state-of-the-art features, including speaker diarization and word-level timestamps.

Contact us
Read more

Speech-To-Text
The evolution and impact of Speech AI: An in-depth conversation with Gladia's CEO Jean-Louis
Once in a while, we like to zoom out of our day-to-day to reflect on the bigger trends affecting our customers to, ultimately, adapt our product accordingly. Today, what are the key shifts happening in voice-first platforms, and how can speech recognition help them to navigate these?
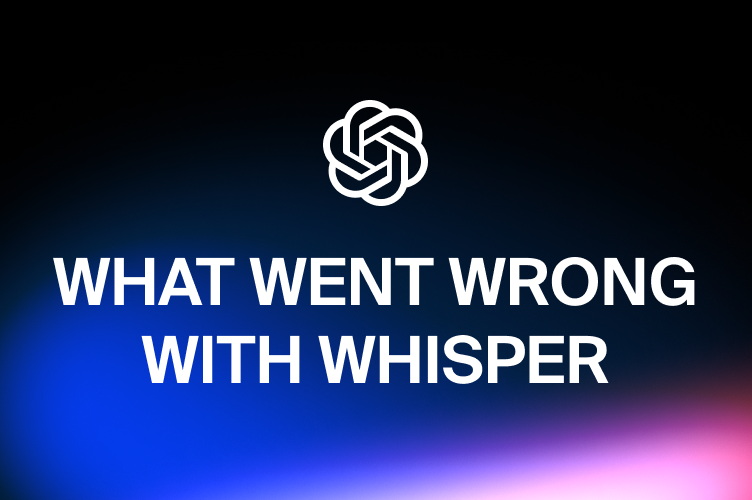
Speech-To-Text
AI Model Biases: What went wrong with Whisper by OpenAI?
When you start working with an AI model, however powerful, you can never be 100% sure of what will happen with it in practice. We've worked with Whisper ASR by OpenAI since its release in 2022 – and what we discovered is nothing short of surprising.

Speech-To-Text
Enhancing CX with AI: Key trends to watch 2024
AI is transforming contact centers at an accelerating pace. Speech AI technologies are at the forefront of this revolution, enabling companies to provide better customer experiences through a combination of advanced agent-assist techniques and fully automated interactions that feel natural and human-like.